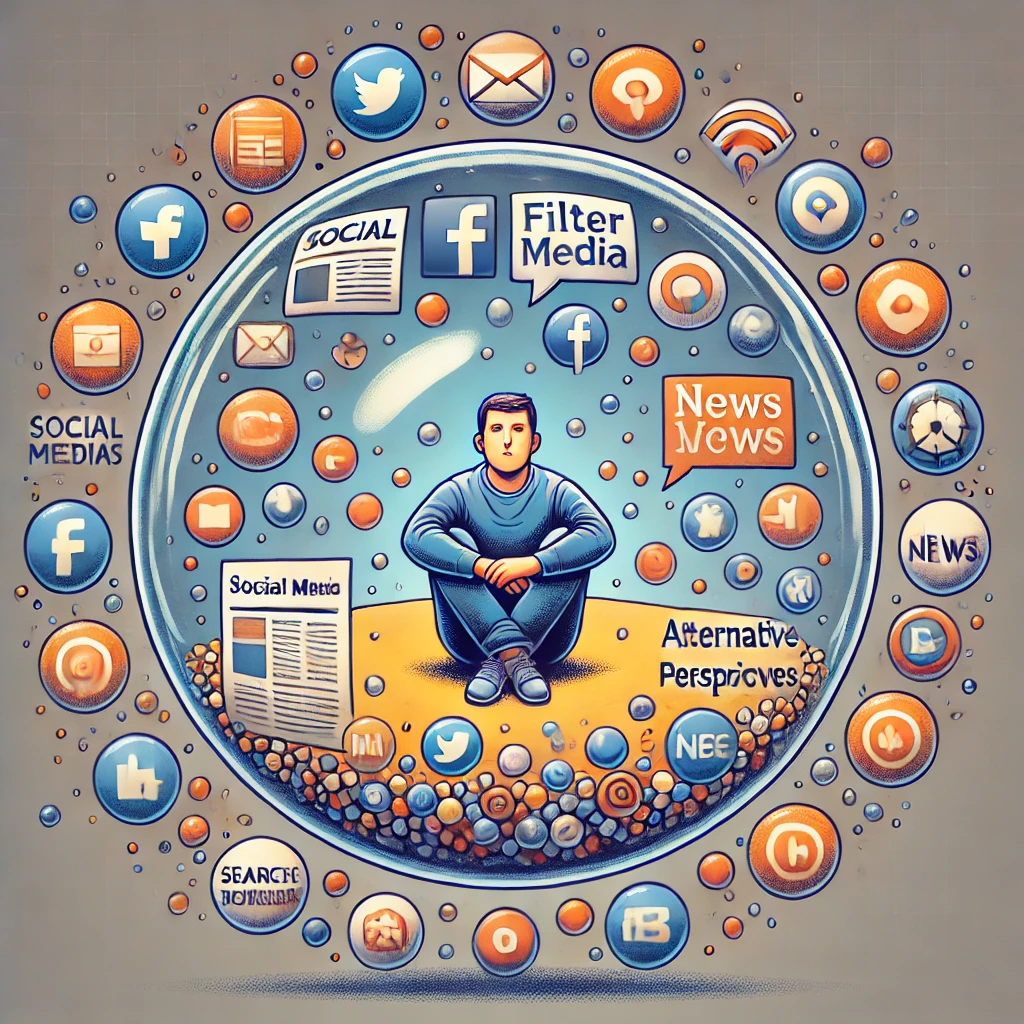
The filter bubble is a concept that describes how algorithms personalise online content based on a user’s past behaviour, creating an environment where people are primarily exposed to information that aligns with their existing beliefs and interests. This happens through search engines, social media platforms, and recommendation systems, which use data such as search history, clicks, and engagement patterns to tailor what a user sees.
Key Aspects of the Filter Bubble:
- Personalisation Algorithms – Websites like Google, Facebook, and YouTube use algorithms to prioritise content likely to engage users, often reinforcing their viewpoints.
- Echo Chambers: Users may become trapped in a cycle where they see only news, opinions, and perspectives that confirm their biases.
- Reduced Exposure to Diverse Views – Because opposing viewpoints are filtered out, users may develop a skewed or one-sided perception of issues.
- Potential for Misinformation – When people are only exposed to like-minded content, misleading or false information can spread quickly.
Example of a Filter Bubble in Action:
- If a person frequently searches for and engages with content about climate change denial, their social media and search engine recommendations may prioritise similar viewpoints while downranking scientific sources that present evidence for climate change.
How to Avoid the Filter Bubble:
- Use private or incognito browsing to reduce algorithmic tracking.
- Follow sources with opposing perspectives to diversify your information.
- Disable or limit personalised recommendations where possible.
- Use neutral or less-biased news aggregators like AllSides or Ground News.
Eli Pariser popularised the term in his 2011 book The Filter Bubble: What the Internet Is Hiding from You. In it, he highlighted concerns about how algorithm-driven content can shape public perception and limit critical thinking.
The filter bubble can significantly affect online investigation results, particularly for professional investigators who rely on search engines, social media, and other online tools to gather intelligence. Here’s how:
1. Skewed Search Engine Results
- Personalised Search Results: Google and other search engines tailor results based on your past searches, location, and browsing history. This can lead to a narrow view of information, potentially missing key data that contradicts prior findings.
- Solution: Use private browsing (Incognito Mode), clear cookies, or search engines like DuckDuckGo that don’t personalise results.
2. Limited Social Media Intelligence (SOCMINT)
- Social media platforms like Facebook, Twitter, and TikTok prioritise content based on user engagement, meaning investigators might only see what aligns with their past activity.
- Some platforms restrict searches based on user preferences, preventing exposure to different communities or hidden threats.
- Solution: Use a fresh or burner account for investigations, avoid interacting with content that could bias future results, and manually search for diverse perspectives.
3. Echo Chambers and Misinformation
- Investigators analysing subjects like political extremism, disinformation campaigns, or fraudulent businesses may fall into algorithm-driven echo chambers.
- If the investigator’s browsing habits lean toward one viewpoint, they might miss counter-narratives or alternative sources.
- Solution: Cross-check sources, use multiple search engines and social media platforms, and manually adjust algorithms (e.g., resetting recommendations on YouTube).
4. Online Ads and False Leads
- Advertisers use profiling to target investigators based on search behaviour.
- If an investigator repeatedly searches for counterfeit goods, for example, they might start seeing misleading ads that appear to be legitimate sources.
- Solution: Use ad blockers, VPNs, or devices to minimise digital footprints.
5. Geolocation Bias
- Results can be geo-restricted, meaning investigators in different locations see different information about the same topic.
- Solution: Use VPNs or manually adjust location settings in search engines.
Key Takeaways for Investigators
- Use multiple tools and search engines to avoid algorithmic bias.
- Clear cookies, use incognito mode, and rotate devices/accounts to minimise personalisation.
- Cross-check information from diverse sources, including international perspectives.
- Be aware of echo chambers, especially in social media-based investigations.
Understanding and mitigating the filter bubble is essential for conducting unbiased, comprehensive online investigations.
Here are some examples illustrating the impact of filter bubbles:
1. Personalised Search Results: Search engines like Google tailor results based on a user’s search history, location, and preferences. This personalisation can lead users to receive information reinforcing their beliefs, potentially limiting exposure to diverse perspectives. For instance, two individuals searching for the same term might obtain different results aligned with their past behaviours.
spreadprivacy.com
2. Social Media Feeds: Platforms like Facebook and Twitter use algorithms to display content that aligns with users’ interests and interactions. This can create echo chambers where users predominantly see posts that confirm their viewpoints, reducing exposure to opposing opinions. For example, users might only see content supporting their preferred candidates during political events, leading to a skewed perception of public opinion.
libraryguides.mdc.edu
3. Online Health Information: When users search for health-related topics, algorithms may prioritise information based on previous searches and clicks. This can result in users encountering information that aligns with their beliefs about health, potentially perpetuating misinformation. For example, someone sceptical about vaccinations might predominantly see anti-vaccine content, reinforcing their stance.
pmc.ncbi.nlm.nih.gov
4. Streaming Services Recommendations: Platforms like Netflix and YouTube recommend content based on users’ viewing history. While this enhances user experience, it can limit exposure to diverse genres or viewpoints. For instance, if a user watches a particular type of content frequently, the platform may continue suggesting similar content, creating a bubble of repetitive information.
nordvpn.com
5. Political Content on TikTok: In the lead-up to the 2024 US presidential election, some TikTok users experienced a filter bubble effect, with their feeds dominated by content supporting their preferred candidate. This created a false sense of widespread support and optimism, shattered when the election results did not align with their expectations. The platform’s algorithm created a bespoke reality, shielding users from opposing views and diminishing their awareness of the broader political landscape.
theverge.com
These examples highlight how filter bubbles can influence perceptions by limiting exposure to diverse information. They also underscore the importance of actively seeking varied perspectives to gain a more balanced understanding of topics.